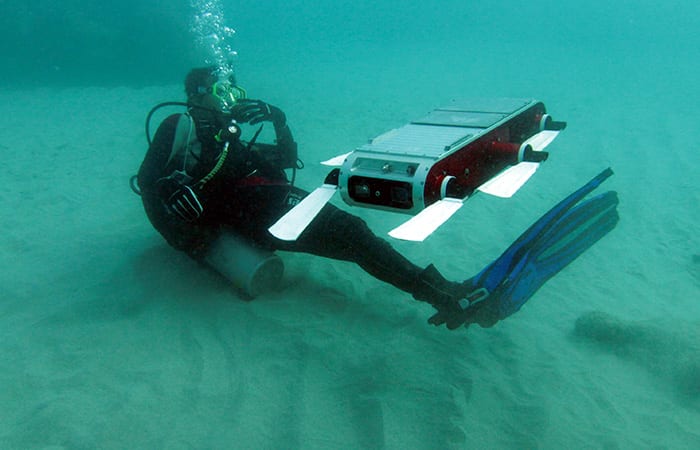
A Smarter Undersea Robot
Engineers seek to correct a curious deficiency
The most sophisticated high-tech deep-diving underwater robot is stymied because it lacks something that even a baby who can’t walk has.
Picture a baby crawling across the floor of an empty room. She roams about for a while, but eventually becomes bored with her surroundings. Then, a few objects are placed on the floor—a shoebox, a plastic cup, maybe a jingling set of keys. The baby’s eyes instantly light up as she moves toward the objects to investigate.
Now picture an underwater robot gliding along a barren stretch of ocean floor. Through its camera’s lens, it “sees” only sand, some pebbles and rocks, and more sand. This goes on and on for a while until, all of a sudden, a few bright-colored fish come into view, and a moment later, a coral reef.
But the robot remains unfazed. Unlike the baby drawn to new and interesting visual stimuli, the robot doesn’t even notice the new objects. It just continues on its pre-programmed mission roaming the seafloor, dutifully collecting reams of images from which scientists might later glean some meaningful discovery.
What is the robot missing?
Curiosity, said Yogesh Girdhar, a postdoctoral scholar with Woods Hole Oceanographic Institution’s (WHOI) Department of Applied Ocean Physics and Engineering.
“We are trying to develop robots that are more human-like in how they explore,” Girdhar said. “With a curious robot, the idea is that you can just let it go, and it will begin to not only collect visual data like traditional underwater robots, but make sense of the data in real time and detect what is interesting or surprising in a particular scene. The technology has the potential to dramatically streamline the way oceanographic research is done.”
Analysis paralysis
Most traditional underwater robots are good at following simple, preset paths to carry out their missions. They typically go up and back through sections of the ocean, tracking a routine pattern that scientists call “mowing the lawn.” Robots that collect images—sometimes hundreds of thousands per mission—don’t have the ability to recognize objects or make decisions based on what they see. That leaves all the analysis and decision-making up to people.
“You can collect all the data you want, but the ocean is too big, and there ends up being way too much data,” Girdhar said. “Human data analysis is very expensive, so this gets in the way of how much of the ocean you can inspect.”
But what if, instead of relying on people to find a needle in a haystack of images, a robot could make independent decisions about what it sees and pinpoint the stuff scientists actually care about?
According to WHOI scientist and engineer Hanumant Singh, it would be a game changer for underwater robotics as we know it today.
“A curiosity-driven robot, in theory, removes the age-old bottleneck we have with image analysis,” Singh said. “Most scientists just throw up their hands with the huge volume of images underwater robots collect, particularly since it can take several minutes to analyze each image. So, they end up only looking at maybe a few hundred, which is a very limited sample size. If we can—even in a small way—have robots autonomously find interesting things and map those versus just being lawnmowers, it could save countless hours of video analysis and give us much more meaningful data to look at.”
Not all scientists, however, subsample their data sets. Tim Shank, a WHOI scientist who uses robots to explore hydrothermal vents and corals in the deep ocean, prefers to have every image looked at by a person despite the amount time and resources it may require.
“In the Gulf of Mexico in 2012, we were trying to determine the footprint of the [Deepwater Horizon] oil spill based on the corals that were impacted,” Shank said. “So we went looking for coral communities and collected at least 110,000 images. We went through those images one by one and scored them based on the presence or absence of geological features and animals. On that cruise, I had four people clicking away on a computer to do the analysis. It was a lot of data.”
Shank feels that while it’s important to err on the side of eyeballing every image in order to capture everything, he admits that having an underwater robot that can explore in a more human-like fashion could lead to making discoveries at a much faster pace.
One fish, two fish
The secret sauce fueling these curiosity-like sensing capabilities is a series of specialized software algorithms Girdhar has developed based on a concept known as semantic modeling. The software enables a robot to build visual models of its surroundings by extracting visual information from objects it sees, recognizing reoccurring patterns, and categorizing those patterns. So, if it finds that rocks and sand usually appear together, the robot will learn the association and may begin to classify that type of scenery as ordinary, paying less attention to it over time. But add something non-routine to the scene—a fish, for example, or something else the robot doesn’t expect to see based its modeled sense of the world—and suddenly the robot will perk up and pay attention.
Once a robot finds something potentially interesting to scientists, it could act on the discovery in a variety of ways. Vehicles remotely operated by pilots in surface ships, for example, could send an “interestingness” signal back to the pilots, who might then reposition the robot closer to the area of interest for a better look. Autonomous robots receiving an “interestingness” signal could modify their own paths to focus on the interesting features and spend time collecting more, higher-resolution information on them.
“We’d like to be able to make robots picky about what they spend time focusing on,” Singh said. “So, for example, it not only pays attention to fish as they pass by the camera but counts them all up and identifies them down to the species level. If a particular scene contains 16 flounder and two rockfish, we want to know that without having to look at thousands of images. We want to make it really simple, like the title of that Dr. Seuss book, One Fish, Two Fish, Red Fish, Blue Fish.”
Proof of concept
Girdhar helped prototype a small and quite curious underwater robot named Aqua as part of his Ph.D. work in Greg Dudek's lab in McGill University in Montreal. Equipped with a wide-angle video camera and six, appendage-like mechanical flippers, the experimental robot was programmed with Girdhar’s specialized algorithms and deployed in the waters off the Bellairs Research Institute in Barbados.
“I went scuba diving with Aqua during that trip, completely unsure of what to expect,” Girdhar said. “We let it go near a coral head surrounded by mountainous sand. It didn’t take long before we noticed that it was more attracted to the coral head. It had very animal-like behavior and started sniffing around like a dog. It didn’t want to go near the sand, since that wasn’t as interesting.”
As he swam around with Aqua, Girdhar himself came into the field view and soon became the singular source of curiosity for the robot. It continued to follow him around until he stopped swimming, at which point the robot simply hovered over him.
“It was very encouraging to see it working the way we had hoped,” he said.
The next frontier
Prototypes are one thing; real-world research tools are another. The next major milestone for the WHOI scientists is to deploy the algorithms on WHOI robots for use in actual research missions.
“If funding permits, we’re hoping to deploy the technology on one of our SeaBED autonomous underwater vehicles (AUVs) in the next few years,” Girdhar said. “Unlike Aqua, which was very small and had relatively poor video resolution, SeaBED will allow us to take advantage of a much larger platform and better camera technology. It will be exciting to see it evolve from just following routine patterns to making its own high-level decisions. It will make the AUV much more autonomous.”
Singh said there’s a big push these days for “scene-understanding” technologies such as semantic modeling, which have already been successfully applied in other areas such as land robotics and visualization of digital photo collections.
“Ten years ago, we were data-poor, and now we’ve become data-rich,” he said. “But it’s impossible to deal with all the data. We don’t care about millions of images, we just want to get the information that’s important to us. The next step for us is to become information-rich, and that’s where things like curious robots come into play.”
This research was funded by the WHOI Postdoctoral Scholar Program, with funding provided by the Devonshire Foundation and the J. Seward Johnson Fund; a Fonds de recherche du Québec – Nature et technologies (FRQNT) postdoctoral research grant; and the Natural Sciences and Engineering Research Council (NSERC) through the NSERC Canadian Field Robotics Network (NCFRN).
Slideshow
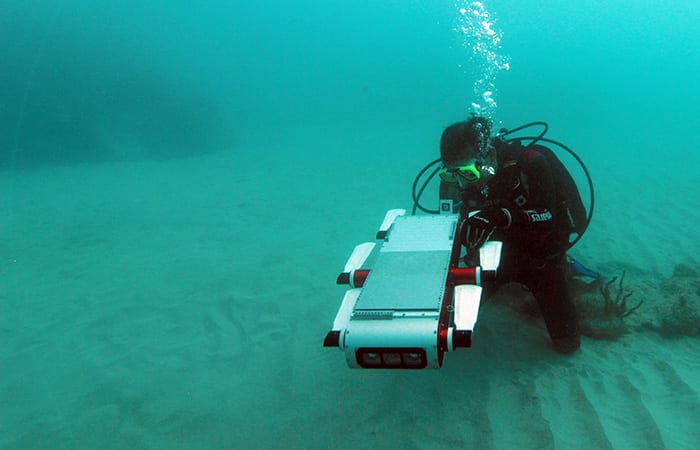
Slideshow
- Yogesh Girdhar helped build an experimental underwater robot named Aqua as a Ph.D. student in Greg Dudek's lab in McGill University in Montreal.
Here, Girdhar sets up Aqua for a mission off Barbados. (Ioannis Rekleitis/McGill University) - Equipped with a wide-angle video camera and six appendage-like mechanical flippers, Aqua was programmed with Girdhar’s specialized algorithms to enable it to be curious—that is, to notice and home in on interesting visual stimuli. (Ioannis Rekleitis/McGill University)
- This image shows the robot’s point of view as it is uses curiosity behavior to explore the ocean. Blue circles mark potentially interesting regions in the robot’s view, and the red circle marks the most interesting thing in its view that attracts the robot’s attention. (Yogesh Girdhar, Woods Hole Oceanographic Institution)
- Soon Girdhar himself came into Aqua's field view
and became the singular source of curiosity for the robot. It continued to follow him around until he stopped swimming, at which point the robot simply hovered over him. (Ioannis Rekleitis/McGill University) - WHOI scientists Yogesh Girdhar, left, and Hanumant Singh would like to take the next step—using the algorithms Girdhar has developed to make robots more curious in larger, better-equipped autonomous underwater vehicles, such as SeaBED (background) developed in Singh's lab. (Sofia Ibarraran)