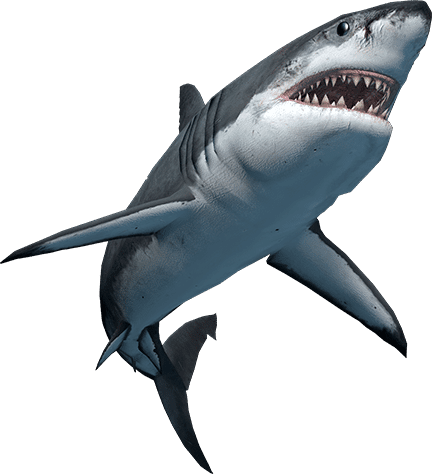
Something fishy is going on.
Your page has not been found.
We're tried everything, but we can't seem to find the page or file that you're looking for. Might we suggest the following solutions?
- If you've typed in the URL yourself, you might want to check your spelling for accuracy.
- If you followed a link from somewhere, let us know so that we can make sure this doesn't happen to another unsuspecting oceanography enthusiast.
If all else fails:
- Try using the search bar at the top of this page.
- Try browsing our top menu. You might find other great items you weren't even looking for.
- Email us. We'd by happy to help you.